Table of Contents
In the world of modern marketing, data-driven decision-making is the key to success. Marketers continually seek ways to optimize campaigns, websites, and product offerings to maximize their effectiveness. One invaluable tool in this quest is A/B testing, a method that allows marketers to compare two versions of a webpage, email, or advertisement to determine which performs better. However, the validity of A/B testing relies heavily on statistical significance, and here’s where economists play a crucial role. In this article, we explore the intersection of A/B testing and statistical significance, highlighting the vital role of economists in validating marketing experiments.
If you’d like to dive deeper into this subject, there’s more to discover on this page: B Practice Tests (1-4) and Final Exams – Introductory Statistics …
Understanding A/B Testing
A/B testing, also known as split testing, involves creating two versions (A and B) of a marketing element, such as a webpage or email, with one key difference between them. This difference could be anything from a headline or call-to-action button to the layout or color scheme. Marketers then randomly assign visitors or recipients to either version A or B to gauge which one performs better in terms of a predefined goal, such as click-through rate, conversion rate, or revenue generated.
A/B testing is a powerful tool for optimizing marketing efforts. It allows marketers to make data-driven decisions by comparing the performance of two or more variations. However, to draw meaningful conclusions, the results must be statistically significant.
If you’d like to dive deeper into this subject, there’s more to discover on this page: Experimentation & Causal Inference
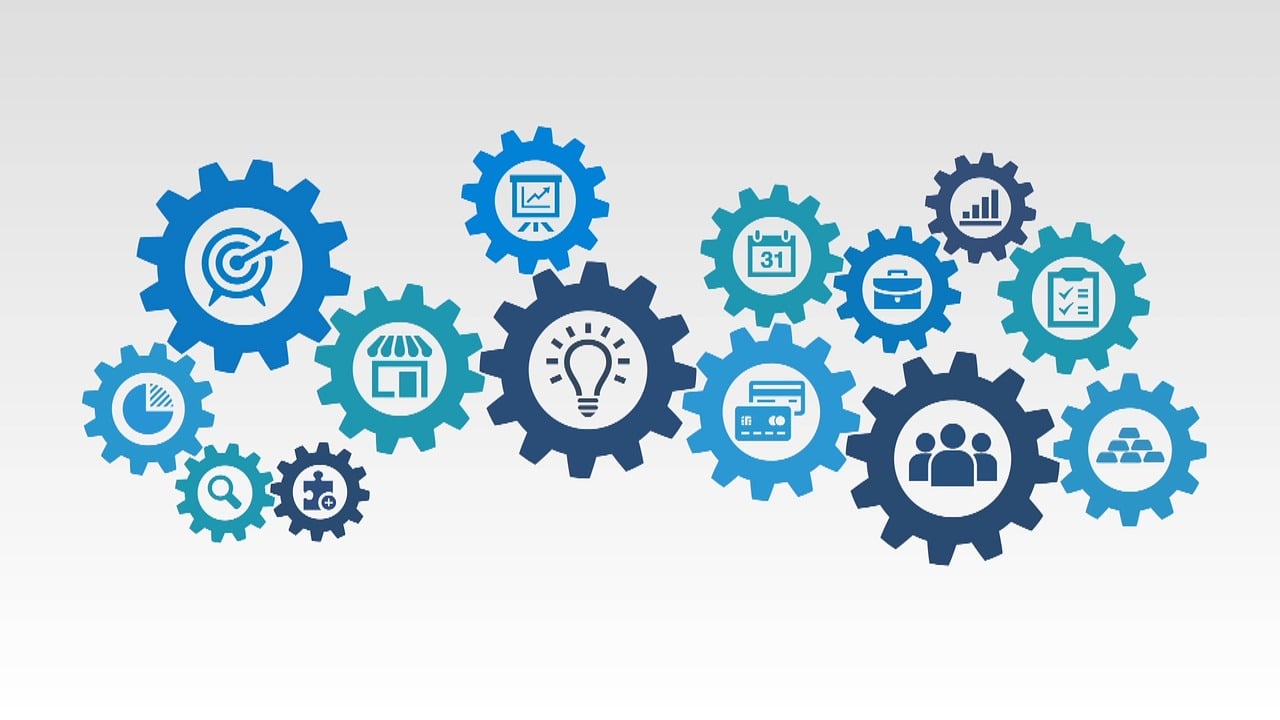
The Role of Statistical Significance
Statistical significance is a critical concept in A/B testing. It indicates whether the differences observed in the test results are likely due to genuine differences between the variations or mere random chance. In other words, it helps determine if the observed effects are reliable and reproducible.
For an A/B test to be considered statistically significant, several factors come into play:
Statistical significance is a pivotal pillar in the world of A/B testing, as it bestows credibility and reliability upon the insights drawn from these experiments. Its role extends beyond a mere binary declaration; it serves as a quantitative measure of the strength and validity of the observed effects. To ascertain statistical significance in A/B testing, several critical factors must be carefully considered:
1. Sample Size: The size of the sample groups in an A/B test plays a fundamental role in determining statistical significance. Larger sample sizes tend to produce more reliable results because they provide greater statistical power to detect meaningful differences. Small samples can yield results that are statistically significant but practically insignificant, underscoring the importance of striking a balance between statistical and practical significance.
2. Effect Size: Effect size quantifies the magnitude of the difference between the variations being tested. While statistical significance tells us if a difference exists, effect size reveals the practical importance of that difference. A small effect size may be statistically significant with a large enough sample but may not have a meaningful impact in the real world.
3. Confidence Level: Statistical significance is often measured at a predefined confidence level, typically 95% or 99%. This indicates the level of certainty that the observed effects are not due to chance. A higher confidence level demands a higher threshold for significance, reducing the likelihood of false positives but potentially increasing the chance of false negatives.
4. P-Value: The p-value is a numerical representation of the likelihood that the observed results occurred by random chance. A low p-value (typically < 0.05) is often considered indicative of statistical significance. However, it’s important to remember that a low p-value alone does not guarantee practical significance or the absence of other confounding factors.
5. Control Group Stability: Ensuring the stability of the control group is crucial. Any external factors or changes that affect the control group but not the experimental group can skew the results. Monitoring and maintaining control group stability throughout the A/B test is essential for accurate interpretation.
6. Duration of the Test: The length of time an A/B test runs can influence its results. Short tests may not capture seasonality or long-term effects, while excessively long tests can be resource-intensive and lead to delayed decision-making.
In summary, statistical significance in A/B testing goes beyond a simple checkbox; it’s a multifaceted concept that demands careful consideration of sample size, effect size, confidence level, p-values, control group stability, and test duration. Balancing these factors ensures that the conclusions drawn from A/B tests are not only statistically significant but also practically meaningful and actionable, providing organizations with the confidence to make informed decisions and refine their strategies.
For additional details, consider exploring the related content available here Channeling Fisher: Randomization Tests and the Statistical …
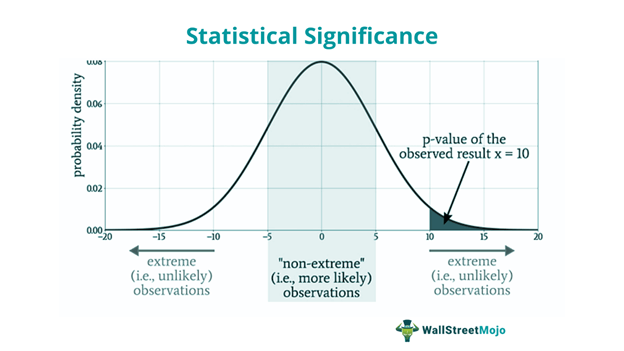
The Economist’s Role in A/B Testing
Economists bring a unique skill set to the world of A/B testing and statistical significance:
nullFor a comprehensive look at this subject, we invite you to read more on this dedicated page: Econometrics: Definition, Models, and Methods

A/B testing is a powerful tool for marketers seeking to optimize their campaigns and strategies. However, the validity and reliability of A/B test results depend on achieving statistical significance. Economists, with their expertise in statistical analysis, sample size determination, and economic perspective, play a vital role in ensuring that A/B tests are designed, executed, and interpreted correctly.
In today’s data-driven marketing landscape, the collaboration between marketers and economists is essential for making sound decisions that drive business growth and profitability. By applying rigorous statistical analysis and economic insights, businesses can extract meaningful insights from A/B tests and confidently implement changes that enhance their marketing performance.
For a comprehensive look at this subject, we invite you to read more on this dedicated page: B Practice Tests (1-4) and Final Exams – Introductory Statistics …
More links
Additionally, you can find further information on this topic by visiting this page: Channeling Fisher: Randomization Tests and the Statistical …